Yannis Angelis
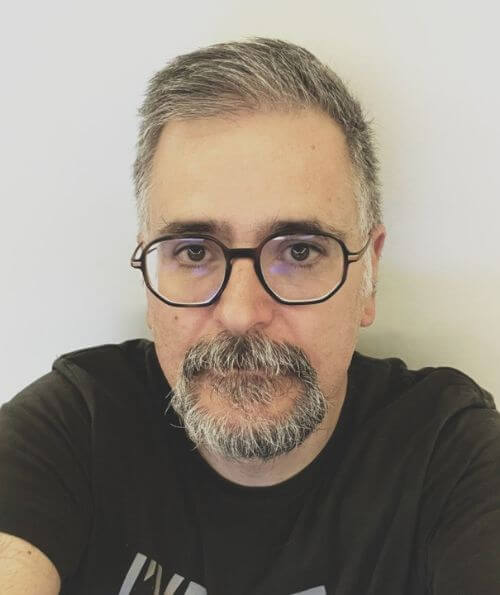
More Sessions From This Speaker
A.I. Summit 2023
November, 2
16:10 -
16:30 EEST
Quick Session
Machine Learning Operationalization at Scale
The international retail company operates in a complex environment, both in terms of technology and organizational structure. This complexity presents significant challenges for data analytics initiatives. Each country within the company creates its own data ecosystem and processes, making it difficult to implement solutions that work across the entire organization.
In this session, we will focus on the "hub and spoke" models that our organization uses to address these challenges in two key areas: technology and product management. Specifically, we will explore the following aspects:
Technology Aspect: We will examine the gaps in data consolidation and homogenization, and discuss the data modelling and data quality framework that has been established to create a common analytics layer. We will also delve into the data engineering framework that supports development at scale and in parallel by multiple vendors, including the Cloud accelerator. Additionally, we will explore the MLOps techniques and guidelines that have been implemented to support the simultaneous lifecycle of models in a large number of countries.
Management Aspect (Cultural): We will discuss the adoption of the SAFe model within the IT department to execute all initiatives, not just those related to AI/ML and Analytics. We will address the project vs. capacity paradox and its consequences, as well as efforts to change the mindset within a traditional organization. Finally, we will share stories from Azure DevOps and other platforms about monitoring and scaling with multi-vendor teams inside the products.
In this session, we will focus on the "hub and spoke" models that our organization uses to address these challenges in two key areas: technology and product management. Specifically, we will explore the following aspects:
Technology Aspect: We will examine the gaps in data consolidation and homogenization, and discuss the data modelling and data quality framework that has been established to create a common analytics layer. We will also delve into the data engineering framework that supports development at scale and in parallel by multiple vendors, including the Cloud accelerator. Additionally, we will explore the MLOps techniques and guidelines that have been implemented to support the simultaneous lifecycle of models in a large number of countries.
Management Aspect (Cultural): We will discuss the adoption of the SAFe model within the IT department to execute all initiatives, not just those related to AI/ML and Analytics. We will address the project vs. capacity paradox and its consequences, as well as efforts to change the mindset within a traditional organization. Finally, we will share stories from Azure DevOps and other platforms about monitoring and scaling with multi-vendor teams inside the products.
Machine Learning
Share this Session